1 Introduction
Much of modern life depends on large organisations. General Electric (GE) makes the engines that power our aircrafts, Johnson & Johnson makes our shampoo, and Google allows us to search the internet. The areas of our lives that are less affected by such private firms depend on public sector organisations such as public hospitals, schools, and police. The justification for the existence of organisations of this size is that the particular combination of individual divisions, alongside a corporate management, will lead to better performance for each of the divisions than they would have been able to generate individually. In other words, the assumption is that such organisations create a synergy—the quality of the whole will be greater than the sum of its parts.
Multi-divisional organisations are typically organised in a hierarchical structure, with a corporate management team and subsidiary divisions. Each division can be made up of several business units. For instance, some of GE’s divisions include GE Aviation and GE Healthcare. Similarly, in the public sector, a hospital system may operate through multiple individual hospitals in different regions.
Such organisations therefore need to make capital allocation decisions. That is, given a limited amount of financial resources, how best to invest in the multiple divisions? Equally? Pick a winner? What metric should be used to compare across divisions? Capital allocation is a critical process to the operation and development of multi-divisional organisations.
The products and services that arise from organisations are necessarily a result of the work of many people. In GE, for instance, the factories that generate aircraft engines need to be staffed by production line workers, accountants are needed for bookkeeping, and software engineers are needed to design and maintain the production systems. Despite this, many important strategic decisions ultimately come from a very small number of people. The decisions that the CEO or other lower level executives make can have large consequences on the life of the company.
It is often assumed that a few people having a lot of decision-making power is for the best. Managers of large organisations often appear to be bold and effective decision-makers. It appears that their position of power and wealth was necessarily arrived at through high competence and rational decision-making, suggesting that the organisation is in good hands. However, there are three reasons why it may be concerning that much of an organisation’s future—and by extension often many more components of the economy—depends on the decisions of a few individual. First, the role of survivorship bias in obtaining the manager’s role is unclear, because the number of managers that used the same management strategy and failed is unknown. Second, decades of research has shown that people’s decision-making is often fallible and that job experience does not always alleviate this fallibility. Third, managers of large organisations often face uncertain environments, which increases the likelihood of managers facing psychological biases.
There are many examples of companies that suffered due to such biases. Overconfidence and confirmation bias likely played a part in Blockbuster’s famous refusal of an offer to buy Netflix in 2000 (Meissner et al., 2015). Further, Roxburgh (2003) identified how Equitable Life Assurance Society unnecessarily anchored on previous interest rate performance and was unprepared when rates changed. In an example of the sunk-cost fallacy, the London Stock Exchange continued investing in an automated settlement system even when it no longer remained profitable. The Bank of England needed to step in and stop the project. Overconfidence in market entry is also a common issue, illustrated by EMI’s introduction into the medical-diagnostics market with the CT scanner (Camerer & Lovallo, 1999; Horn et al., 2005). By underestimating the competition and overestimating their own capabilities they eventually incurred losses and exited the market.
One class of biases has not been well studied: capital allocation biases. While some previous work investigating these biases exists (e.g., Bardolet et al., 2011), many questions still remain unanswered. This is a rather large hole in the literature because capital allocation decisions are at the centre of executive and lower level managers’ roles. When making capital allocation decisions, there are elements of the decision-making environment that can be deceiving for managers. This thesis examines how the framing of a series of business projects affects people’s decisions about those projects. Specifically, the same set of projects, presented in aggregate form, is much more likely to be accepted. Further, sometimes people are distracted by extraneous semantic information, such as the relative similarity of the options.
The results of the thesis show that although people in general make sensible decisions, they fail to use critical information to inform their decisions. Specifically, information about metric variance is ignored even when other metrics are available. Further, people seem to appropriately use statistical and anecdotal information based on relevance to the situation at hand, but ignore information about the sampling of the anecdote. Not appropriately using these kinds of statistical concepts has important financial consequences, discussed below.
All the experiments in the thesis use laypeople, except for one experiment. However, past work generally shows the same biases in managers and laypeople (with some showing more bias in managers, e.g., Haigh & List, 2005). Further, upcoming studies will directly test managers to determine any potential expertise effects.
Section 1.1 will explain how the capital allocation process functions in hierarchical organisations and why it is necessary to analyse such a process with a psychological approach. Section 1.2 reviews the literature on decision-making biases and how these may apply to capital allocation decisions. Section 1.3 will then summarise the rest of the thesis chapters.
1.1 Capital Allocation in Hierarchical Organisations
The purpose of a multi-divisional organisation is to generate more value than any of the individual divisions combined. The whole should be greater than the sum of its parts. Previous work suggests that this is achieved due to factors such as reduced transaction costs (Coase, 1937; Liebeskind, 2000; Teece, 1982, 1980; Williamson, 1981), shared resources (Barney, 1991; Wernerfelt, 1984), increased competitive advantage (Porter, 1980, 1985), increased monitoring (Gertner et al., 1994), and increased synergies (Barney, 1988). The underlying logic is the same: a multi-divisional organisation will be successful if it manages its divisions using processes and resources that are shared or, better yet, are complementary.
In order to successfully manage multiple units, large organisations developed a hierarchical structure. Bower (1970) identified three levels of the typical management hierarchy: business, division, and corporate. These are equivalent to front-line (or bottom), middle, and top level managers (Noda & Bower, 1996). Early theorists suggested that the strategy for the organisation’s growth is driven completely by the top managers; the rest of the organisation simply enacts their proposals. However, Mintzberg and Waters (1985) emphasised the role of an emergent strategy, in which lower level managers affect change in the organisation’s strategy. Other work proposed and found evidence for an iterated process in which a strategic context may be set by top managers, but business projects advanced by lower level managers also contribute to driving the strategy of the organisation (Bower, 1970; Burgelman, 1983; Noda & Bower, 1996).
The way that capital is allocated in an organisation is very important to its growth and longevity. This process is a part of the broader process of resource allocation. A resource can refer to many types of assets that an organisation owns, both tangible and intangible, of which capital is only one (Wernerfelt, 1984). The capital allocation process itself is an important driver of the strategic outcomes of an organisation (Bower, 1970; Bower & Gilbert, 2005), and as a result, is an important influence on its financial performance (e.g., Arrfelt et al., 2015; Bardolet et al., 2010). Sengul et al. (2019, p. 72) describe intra-firm capital allocation as “(i) a process of determination, comparison, and selection among multiple investment alternatives, (ii) taking place across organizational levels of the firm, and (iii) influenced and constrained by the external context in which the firm is situated.” In capital allocation, business-level managers typically formulate project proposals, which their division managers then evaluate. The division managers then choose the projects to send for final approval with the corporate managers. The supply of available capital is also influenced by external sources such as investors, competitors, and customers. However, this thesis focuses on the comparison and selection processes that are relevant during business project evaluation.
Managers ultimately have only limited information about the projects that they evaluate. They typically have access to descriptive information about the investment and its known properties, but also are provided with financial metrics that estimate the returns on the investment. There are many such metrics; they usually attempt to encapsulate a trade-off between predicted future gains, present losses (in the form of the capital spent to pay for the investment), and opportunity costs. Examples include net present value (NPV), internal rate of return, return on investment, cost-benefit, and pay-back period. This thesis focuses on NPV, since it is one of the most frequently used metrics for project evaluation (Graham et al., 2015; Graham & Harvey, 2001; Remer et al., 1993). NPV is the difference between the money that a project is forecasted to make and the initial investment in its development (accounting for the time value of money), as shown in Equation (1.1):
\[\begin{equation} \text{NPV}=\sum_{t=0}^n \frac{R_t}{(1+i)^t}, \tag{1.1} \end{equation}\]
where \(t\) is the time of the cash flow, \(i\) is the discount rate, \(R_t\) is the net cash flow, and \(n\) is the total number of periods. NPV is a useful metric because simply knowing that it is positive suggests that the project that it describes should be profitable. Therefore, metrics such as these have a strong influence on the decision of the manager evaluating a project.
However, there are other influences on project evaluations other than the value of the financial metrics. For instance, politics within or outside the company can lead to situations in which a decision is based on social influence or even manipulation (Garbuio & Lovallo, 2017). Such influence is not necessarily negative; it may involve qualitative feedback from, for instance, a more senior manager (Thamhain, 2014). Research has also shown that the media can have a tangible influence on managerial decision-making (Bednar et al., 2013; Liu & McConnell, 2013). Other sources of influence are the organisational structures and incentives that are in place both externally (Kokkinis, 2019) and internally to the organisation (Rajan et al., 2000; Ullrich & Tuttle, 2004). Such dynamics have also been the subject of economic modelling investigations (Cavagnac, 2005; Ortner et al., 2017; Reichelstein, 1997). Project proposals might also be affected by certain approval structures. For instance, managers might submit overly-optimistic project proposals if they know that the corporate team only accepts projects with a certain minimum NPV forecast.
Another potential organisational influence on capital allocation is the extent of diversification present in an organisation. A diversified organisation is one that possesses different divisions that are unrelated in some way. Penrose (1959/2009, p. 96) defined it as such:
a firm diversifies its productive activities whenever, without entirely abandoning its old lines of product, it embarks upon the production of new products, including intermediate products, which are sufficiently different from the other products it produces to imply some significant difference in the firm’s production or distribution programmes.
Previous work found that organisations that are made up of more related divisions are more successful than those that are made up of unrelated divisions (Harrison et al., 1993; Rumelt, 1974; Shelton, 1988; Wernerfelt & Montgomery, 1988). This is also true within business divisions (Davis et al., 1992). However, more diversified firms have also been shown to be associated with profitability (Grant & Jammine, 1988). This is usually explained by the ability for such firms to avoid risk associated with any one market. Some of the discrepancy in diversification findings has been explained to be due to the specific measures used (Lubatkin & Shrieves, 1986). It may also be because most studies used Standard Industrial Classification (SIC) codes to measure diversification (e.g., Rumelt, 1974), whereas others operationalised it using other approaches (e.g., resource-based; Harrison et al., 1993).
The advantage that related organisations have had has been explained through synergies (Barney, 1988). That is, an organisation with two divisions that can use their resources to better one another are better off together than separately. The 1960s saw a general rise in mergers and acquisitions from executives seeking to diversify their organisations. However, doing so simply for the sake of increasing divisions, rather than an understanding of the possible synergies, leads to the organisation actually being worth less than the sum of its parts (known as a diversification discount; Lang & Stulz, 1994). In fact, many organisations that acquired other businesses to diversify subsequently end up divesting them (Porter, 1987). For instance, in 2018 Australian conglomerate Wesfarmers demerged its Coles division, a successful retailer. Since then, the share price for both companies has risen by approximately 62% and 32%, respectively (Boyd, 2021).
While much of the performance of an organisation depends on influences that are external to the individual managers (e.g., organisational, political), psychological factors are often also quite consequential. For instance, on the one hand, organisational factors such as relevant support teams and approval processes may influence capital allocation depending on the extent of an organisation’s extent of diversification. On the other hand, psychological factors such as ability of managers to compare between business project proposals may also impact allocation differently depending on the organisation’s diversification. It is likely to be more difficult for a manager to evaluate project proposals from two dissimilar divisions that it is to evaluate those from two similar divisions. The organisational influences discussed above often assume that the manager that is making the decisions acts rationally, as per traditional economic theory. However, surveys of executives show that CEOs and CFOs often rely on non-financial factors for capital allocation decisions (Graham et al., 2015). Executives in these surveys identified manager reputation and confidence as two of the most important factors for capital allocation decisions. Further, research in psychology has shown that cognitive biases can influence such capital allocation decisions. Section 1.2 discusses such biases and the relevant implications for the thesis.
1.2 The Psychology of Capital Allocation
Managers of large organisations are generally assumed to have a superior decision-making capability compared with non-managers. However, managerial decision-making involves many of the same processes that have been shown to be affected by psychological biases in the general population (Das & Teng, 1999; McCray et al., 2002; Schwenk, 1984). Further, an organisation’s success ultimately depends on strategic decisions made by top level managers (Mazzolini, 1981). Therefore, despite early work attempting to analyse such decisions using a structured organisational analysis (e.g., Mintzberg et al., 1976), it is important to understand the potential influence of psychological biases on managerial decisions. Research in the field of behavioural strategy has started to do this (Powell et al., 2011).
Psychological research has shown that people tend to make decisions that are inconsistent with neoclassical economic theory. For instance, expected utility theory (EUT; Friedman & Savage, 1948; von Neumann et al., 1944) assumed that people have complete information when making decisions. However, both laypeople and managers of organisations are limited in the amount of information that they have and their ability to use it (Cyert et al., 1956; Simon, 1955). Such inconsistencies with economic prescription are likely to have evolutionary origins, so are sure to be adaptive in certain environments (Bettis, 2017; Gigerenzer, 2008; Haselton et al., 2009). However, there are many situations in which such inconsistency with economic theory can have bad consequences.
Research has shown many ways in which the allocation of capital in an organisation can be influenced by psychological biases. For instance, Benartzi and Thaler (2001) found that people tend to allocate their retirement fund equally between the available options, regardless of their composition. This naive diversification bias was also found in capital allocation for hierarchical firms (Bardolet et al., 2011). Managers allocated capital equally across the available divisions in the firm, regardless of performance. Analysis of real companies found that this behaviour is damaging to firm performance because it means that lower performing business units get subsidised by higher performing units, which are not operating at their full potential (Arrfelt et al., 2015; Bardolet et al., 2010). Subsequent studies found that business unit size also matters; capital allocation to both the smallest and largest units is disproportionate to their actual profitability levels (Bardolet et al., 2017). This was attributed to a combination of naive diversification and political power effects.
Relatedly, people tend to continue expending capital into investments that appear to be failing (Staw, 1981). This escalating commitment is another way that psychological biases can influence allocation in an organisation. This pattern of decision-making is likely a consequence of the sunk cost fallacy, in which people avoid “cutting their losses” even when they know that they cannot recuperate an investment (Parayre, 1995).
Managers also do not always seem to seek profit maximisation. Shapira and Shaver (2014) offered managers and Master of Business Administration (MBA) students two investments from a hypothetical firm: one with the same expected returns as the average of the firm’s current investments and one with lower returns than the firm’s average returns. However, both investments were profitable, so to maximise firm profits both should be chosen. Instead, participants were more likely to only choose the first investment. It seems that the firm’s average returns served as an anchor, so participants did not want to reduce the firm’s average returns, regardless of profitability.
The way that information is presented can also influence allocations. For instance, Yates et al. (1978) showed that people’s evaluations are sensitive to the level of detail in the information provided. They found that people devalued descriptions of university courses more when they had less detail. This may be relevant for managers evaluating project proposals. A proposal might appear more attractive simply due to the level of detail in it, even if the level of detail does not correspond with the quality of each proposal.
Further, people tend to be over-confident in their decisions and forecasts. This has been shown in laypeople (Langer, 1975; Mannes & Moore, 2013; Puri & Robinson, 2007; Soll & Klayman, 2004), as well as in IT professionals (McKenzie et al., 2008) and managers (Barone-Adesi et al., 2013; Kahneman & Lovallo, 1993; Lovallo & Kahneman, 2003). This is important for higher-level managers that evaluate project proposals because the metrics that rely on forecast estimates may be biased by the over-confidence of the lower-level manager that created the proposal. Further, the higher-level manager evaluating the proposal may in turn be over-confident about its prospects due to factors that are unrelated to the underlying value. Overconfidence is also seen when considering the success of projects in hindsight (Bukszar & Connolly, 1988; Christensen-Szalanski & Willham, 1991). This means that it is less likely that managers will be able to effectively learn from both past mistakes and successes due to the potentially erroneous belief that the outcome was anticipated.
Managers often create sensitivity analyses, estimating the worst case, best case, and most likely scenario for a forecast. However, these are likely to be anchored on past experiences that further the manager’s existing beliefs. In fact, prior research has shown that people are poor at constructing subjective probability distributions (e.g., Alpert & Raiffa, 1982; Schaefer & Borcherding, 1973; Tversky & Kahneman, 1974; von Holstein, 1971). Therefore, this suggests that even if the lower-level managers that construct project proposals calibrate their forecasts so that they are not over-confident, they are still likely to provide inaccurate estimates of their degree of confidence.
The above summarises many of the currently known psychological biases related to capital allocation. This thesis focuses on three essential processes within the capital allocation process: (a) risky choice, (b) the comparison between diversified businesses, and (c) the influence of prior experience. Each of these is prone to separate biases, that are also interrelated. The subsequent subsections review the literature for these processes.
1.2.1 Risky Choice
Neoclassical theories such as EUT suggest that when faced with multiple risky options people should choose the option with the highest expected value (EV), all else being equal. This means multiplying the value of each option by its probability and comparing the resulting values (first documented in Pascal, 1670/1999). For instance, imagine being presented with the following two choices:
a gamble that involves a 50% chance gaining $200 and a 50% chance of losing $100; or
gaining/losing nothing.
In option A, the EV is calculated as \(200 \cdot 0.5 - 100 \cdot 0.5 = 50\). Since the EV for option A (50) is higher than the EV for option B (0), EUT would suggest that option A should be chosen.
This basic principle was extended by Bernoulli (1738/1954), who suggested that a person’s subjective value of money differs depending on their current wealth. This diminishing marginal utility suggests that the more money a person already has, the less value acquiring more money will have for him. For example, the experience of a rich man that finds $10 on the street is very different to the experience of a homeless man that finds $10 (Bradley, 2013). Even though $10 was gained in both cases, $10 has less value to a person that already has, for example, $1,000, than for a person that initially only has $10. This principle is usually modelled as an power function (with a fractional exponent).
Prospect theory (Kahneman & Tversky, 1979; Tversky & Kahneman, 1992) challenged EUT by suggesting that people’s subjective value of money does not depend on their state of wealth—it depends on a change of wealth from a reference point. This is important because people’s subjective value of money is different depending if they are gaining or losing money. Specifically, losses have a stronger psychological impact than equivalent gains. This disparity is one of the most settled and consistent findings in psychology and economics, having been well-replicated (e.g, Ruggeri et al., 2020). The fact that losses loom more than equivalent gains for the vast majority of people is referred to as loss aversion (Kahneman & Tversky, 1979). This finding was the primary reason that Daniel Kahneman won the Nobel Prize in Economics in 2002 (Kahneman, 2003). Loss aversion has been found with small amounts of money in experimental settings (Kahneman & Tversky, 1979; Tversky & Kahneman, 1992) and with millions of dollars in corporate settings (Koller et al., 2012; Swalm, 1966). The effect has been found in young children (Harbaugh et al., 2001), the numerous disparate cultures in which it has been tested (Weber & Hsee, 1998), and even in capuchin monkeys (Chen et al., 2006). Furthermore, a neural basis for loss aversion was identified (Tom et al., 2007). Therefore, loss aversion is clearly central to human cognition and behaviour.
The function that represents the value of a prospect describes both loss aversion and diminishing marginal utility, as shown in Equation (1.2):
\[\begin{equation} v(x) = \left\{ \begin{array}{l} x^\alpha \text{ if } x \ge 0 \\ -\lambda(-x)^\beta \text{ if } x < 0, \tag{1.2} \end{array} \right. \end{equation}\]
where \(x\) is the possible outcome, \(\lambda\) represents the loss aversion coefficient, and \(\alpha\) and \(\beta\) represent the diminishing marginal utility for gains and losses, respectively.
In other words, loss aversion means that losses have more impact than equivalent gains. In fact, the impact of loss aversion can be expressed even more precisely, as a measurement of the ratio of the slopes of the curve for gains and losses. This measure tells us the average amount that losses have more impact than equivalent gains. In a sequel to the original prospect theory paper, Tversky and Kahneman (1992) measured a median coefficient (\(\lambda\)) of 2.25 of loss aversion. This means that people respond to losses 2.25 times more than equivalent gains. Similarly, this paper measured a median exponent (representing diminishing marginal utility, \(\alpha\) and \(\beta\)) of 0.88 for both gains and losses. This means that people discount money the more of it they have by a rate of \(x^{0.88}\).
Figure 1.1 shows loss aversion as the function being steeper in the domain of losses than the domain of gains. It shows diminishing marginal utility by the slight curve of the function. Equivalent changes in actual wealth from the references point (x-axis) have different impacts on the changes’ subjective value (y-axis). An increase in wealth (\(x = 1\)) brings about an equivalent increase of value (\(y = 1^{0.88} = 1\)). However, a decrease in the same amount of wealth (\(x = -1\)) brings about a decrease in value 2.25 times the value of the equivalent gain (\(y = -2.25 \cdot (-(-1))^{0.88} = -2.25\)).
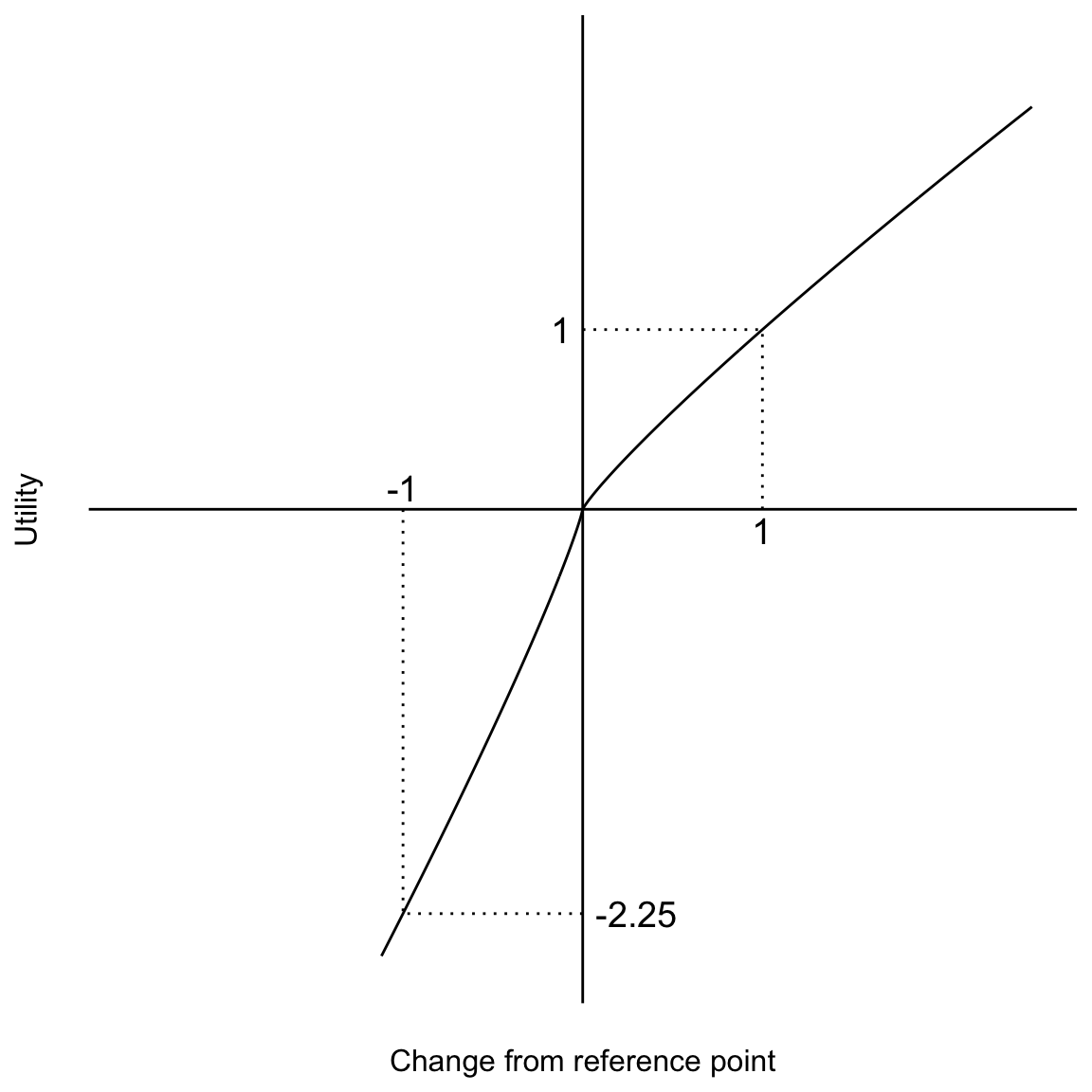
Figure 1.1: An example of the value function in prospect theory.
This research is relevant to capital allocation because the project proposals that managers evaluate invariably involve an element of risk. Therefore, managers are likely to be affected by similar effects on risk that have been shown in laypeople. However, hierarchical organisations offer an even more complex situation. Lovallo et al. (2020) found that the risk profiles of lower-level managers are lower than those of the top managers. They suggest that this may be due to lower-level managers’ loss aversion to accepting projects that may jeopardise their job. However, the top managers recognise that a loss in one or more business units is likely to be offset by gains in other units. Such an inconsistency in risk profiles across the levels of an hierarchical organisation fails to take advantage of the benefits of risk aggregation, which has long been understood in external markets (Markowitz, 1952).
Lovallo et al. (2020) suggested that lower-level managers’ failure to aggregate risk to the degree desired by top executives is costing companies approximately a third of the total EV of new project proposals. This is an example of a negative consequence associated with ignoring statistical concepts such as risk aggregation. It is thus critical to identify ways to support risk aggregation across organisational hierarchies. The psychological literature shows that people’s risk aggregation is facilitated through various choice bracketing manipulations. However, there has been no work that investigated such situations without providing participants with feedback in between decisions; this critically limits the external validity of this work because in the real world, organisations evaluate several projects before seeing the outcomes of any one decision. The experiments presented in Chapter 2 investigate the effects of choice bracketing on risk aggregation without feedback.
1.2.2 Project Similarity
When evaluating project proposals, managers are likely to be influenced by the relative similarity of the available options to each other. The extent to which this may be true is important especially since the increase firm diversification. Organisations are not only varied by the number of divisions which they possess but also by the extent of diversification. This means that managers are likely to find themselves comparing across dissimilar types of projects.
As mentioned above, there are likely many organisational and financial reasons why the extent of diversification in an organisation would impact its performance. However, the impact of psychological factors has not been investigated. Specifically, project similarity, which is an organisational factor, is likely to affect the project comparison process, which is a psychological factor. This may then have downstream consequences on firm performance through, for instance, the kinds of financial metrics that are used and how they are evaluated. Having more similar projects to compare may mean more attributes on which to evaluate, whereas a dissimilar comparison may lead to a situation in which a manager has to rely on potentially unreliable metrics.
Structure-mapping theory (Gentner, 1983; Gentner & Markman, 1997) provides a model of comparison that psychologically distinguishes similar and dissimilar allocation tasks. This framework models comparison as a process of bringing conceptual structures into alignment which, when possible, puts shared dimensions into correspondence. Alignment both highlights when two conceptual structures share dimensions, but also highlights how the two structures differ along those shared dimensions, called alignable differences. For example, when comparing two oil discovery projects, all the relevant processes of planning an exploration and measuring the amount of hydrocarbons in a prospect might be identical, but the specific amount measured will be different. This is the alignable difference: a difference between the two projects that is constrained within the same conceptual structure. However, when comparing between an oil field and a refinery, there will be significantly more non-alignable differences, because the two domains do not share component dimensions. That is, many of the processes that exist in the exploration business unit have a significantly different dimensional structure to those in the refinery business unit, such that it will be difficult to find meaningful alignments. More non-alignable differences mean that there are less opportunities to make meaningful comparisons, and so would make predicting relative project success and ranking their priority more difficult. Chapter 4 experimentally examines business project comparisons and how project alignment affects capital allocation decisions.
When evaluating projects, managers make use of financial metrics, such as NPV. However, such metrics are reliant on forecast estimates of, for instance, future cash flows. Do managers take into account such inherent variance in their decisions? This is especially important to investigate given the above discussion. In cases of non-alignable comparison managers may rely on a potentially unreliable metric. On the other hand, in an alignable comparison, managers might have the option to based their decisions on the relative reliability of different metrics. It is important to remember that all such decisions are often very consequential for the manager. That is, the project could ultimately make the company money and lead to future opportunities for the manager, or potentially cause financial harm to the company (and subsequently lead to a job loss). This is another example of the way in which ignoring certain statistical concepts—here metric variance—can have negative consequences for an organisation.
Psychological research shows that laypeople are in general quite poor at using numerical variance information (Batteux et al., 2020; Galesic & Garcia-Retamero, 2010; Konold et al., 1993; Vivalt & Coville, 2021). However, it is unclear to what extent managers would be sensitive to variance information in the metrics associated with the projects that they evaluate. On the one hand, perhaps managers’ financial training will allow a consideration of such variance estimates, but this might not manifest in a situation in which managers have already been shown to be prone to biases. Chapter 4 investigates whether participants are as sensitive to verbally-instructed reliability information as they are to numerical reliability information.
1.2.3 Reasoning From Past Cases
Managers often use past events to reason and make predictions about the future (Einhorn & Hogarth, 1987). Such past events may be those that happened to the individual manager, a case from the organisation’s history, or from an external source. This will especially be the case in a project evaluation scenario when a given project is hard to compare with the other projects at hand. However, managers evaluating project proposals may make inappropriate comparisons when considering the target project to other cases. For instance, people tend to limit the size of the comparison set to a small number. This is often only a handful of cases, or even one. Doing this might mean only considering potentially irrelevant surface similarity to the current situation and not aligning the underlying causal structure. Further, this might mean not considering other similar projects.
Tversky and Kahneman (1974) discussed a number of biases that may influence such processes. The availability bias is seen when people mistake the ease of retrieval of information for its frequency. Further, research on analogical retrieval showed that people are more likely to retrieve surface similar cases than those with a relational connection (Gentner et al., 1993). As such, managers are likely to recall cases that may not be sufficiently relevant to their target situation and be overly-confident about the frequency of such cases occurring. Such a focus on a particular case might then also lead to an anchoring effect, wherein other decisions might be disproportionately seen as relevant. Tversky and Kahneman (1974) also found that people are not sensitive to properties of sample size such as the greater amount of non-representative outcomes in small samples. This means that managers are even less likely to appreciate the importance of considering a large sample of cases when drawing conclusions to a target problem. Tversky and Kahneman (1974) also note an insensitivity to predictability, in which people do not take into account the reliability of the information that they have to make a prediction. This might mean that managers may struggle to ideally weigh evidence of varying degrees of reliability.
External sources that may be used to compare to a target situation include business case studies. Considering such examples of prior business decisions or events are the way that most MBA students learn about the business world. Publications such as Forbes or Harvard Business Review publicise various businesses’ successes and failures and so may create an allure to use such case studies in the decision-making process. On the other hand, managers may have access to more aggregated data about their industry from, for instance, consultancy companies. How do managers use these various types of evidence in their decision-making?
Research on this topic suggests that managers tend to prefer anecdotes over statistics, unless aided (Wainberg, 2018). This is a concern because Gavetti et al. (2005) suggests that managers often make use of case studies quite poorly. The analogy literature draws a distinction between surface similarity, in which a mapping is made between easily identifiable but potentially functionally irrelevant attributes, and relational similarity, in which the underlying mechanism is considered. Are managers sensitive to the deeper causal mechanisms that underlie the anecdotes they judge? Or are they simply influenced by surface similarity? Chapter 6 investigates the extent to which people use anecdotes or aggregated data based on the relevance of the anecdote to the target project during capital allocation. It also considers whether people are sensitive to information about the distribution from which the anecdote was sampled. Ignoring this statistical concept can have negative consequences for an organisation by potentially over- or under-estimating the relevance of a past case and therefore making an ill-informed investment.
1.3 Chapter Overview
In sum, the potential consequences of a diversified hierarchical structure are that business projects will be considered one at a time, and if they are considered together, disparate project types will make comparisons hard. Considering projects one by one might mean that risk is not aggregated across projects and therefore value is lost. The difficulty to compare will lead to both potentially relying on unreliable metrics, and relying on improper anecdotal evidence. The thesis is that people often go half-way. They do not completely disregard the normative strategy, but also struggle to use statistical concepts such as aggregation, variance, and sampling.
The previous section identified three capital allocation processes that are currently under-studied and so are important to investigate further. First, the evaluation of individual project proposals may lead to managers only considering such projects one at a time, despite the opportunity of aggregating a portfolio of such projects. The choice bracketing literature suggests that there are ways of facilitating such aggregation, but does not investigate this without providing participants inter-trial feedback. Second, in situations in which managers compare multiple projects, the structural alignment literature suggests that managers in diversified firms will struggle to allocate capital, more than those in more integrated firms. Further, these managers may not be sensitive to the variance inherent in the financial metrics they rely on. Third, a difficulty to compare across existing projects may instead mean a reliance on prior case studies from personal or external experience. Research on anecdotal bias suggests that managers may rely more on such case studies than on aggregated data, but it is unclear whether their decisions will depend on anecdote relevance. Further, it is unclear if they will appropriately use information about the anecdote’s sample distribution.
The rest of this thesis investigates the psychology of capital allocation decisions in three chapters that describe empirical work, two theoretical chapters, and a general discussion chapter. Chapter 2 describes two experiments that investigate the effects of choice bracketing on risk aggregation without feedback. Chapter 3 is a short theoretical chapter that discusses the difference between evaluating project proposals with inherent budget estimates and the process of allocating an existing budget top-down. Chapter 4 describes three experiments that investigate the effects of alignment and reliability type—verbal or numerical—on allocations. Chapter 5 is another short theoretical chapter that discusses the trade-offs that people make when using information to evaluate project proposal options. Chapter 6 describes two experiments that investigate the effects of anecdote similarity on the anecdotal bias. Finally, Chapter 4.2.3 discusses the theoretical and practical implications of the empirical chapters and concludes the thesis.
References
Alpert, M., & Raiffa, H. (1982). A progress report on the training of probability assessors. In D. Kahneman, P. Slovic, & A. Tversky (Eds.), Judgment under uncertainty (pp. 294–305). Cambridge University Press. https://doi.org/10.1017/CBO9780511809477.022
Arrfelt, M., Wiseman, R. M., McNamara, G., & Hult, G. T. M. (2015). Examining a key corporate role: The influence of capital allocation competency on business unit performance. Strategic Management Journal, 36(7), 1017–1034. https://doi.org/10/gkhhc5
Bardolet, D., Brown, A., & Lovallo, D. (2017). The Effects of Relative Size, Profitability, and Growth on Corporate Capital Allocations. Journal of Management, 43(8), 2469–2496. https://doi.org/10/gjvc4v
Bardolet, D., Fox, C. R., & Lovallo, D. (2011). Corporate capital allocation: A behavioral perspective. Strategic Management Journal, 32(13), 1465–1483. https://doi.org/10/cn6xsb
Bardolet, D., Lovallo, D., & Rumelt, R. (2010). The hand of corporate management in capital allocations: Patterns of investment in multi- and single-business firms. Industrial and Corporate Change, 19(2), 591–612. https://doi.org/10/ctxs4t
Barney, J. B. (1988). Returns to bidding firms in mergers and acquisitions: Reconsidering the relatedness hypothesis. Strategic Management Journal, 9(S1), 71–78. https://doi.org/10/dmpq6x
Barney, J. B. (1991). Firm Resources and Sustained Competitive Advantage. Journal of Management, 17(1), 99–120. https://doi.org/10/gpm
Barone-Adesi, G., Mancini, L., & Shefrin, H. (2013). A Tale of Two Investors: Estimating Optimism and Overconfidence. http://www.ssrn.com/abstract=2319260
Batteux, E., Bilovich, A., Johnson, S., & Tuckett, D. (2020). Impressed by Numbers: The Extent to Which Novice Investors Favor Precise Numerical Information in a Context of Uncertainty (SSRN Scholarly Paper ID 3595409). Social Science Research Network. https://doi.org/10.2139/ssrn.3595409
Bednar, M. K., Boivie, S., & Prince, N. R. (2013). Burr Under the Saddle: How Media Coverage Influences Strategic Change. Organization Science, 24(3), 910–925. https://doi.org/10/gf33wh
Benartzi, S., & Thaler, R. H. (2001). Naive Diversification Strategies in Defined Contribution Saving Plans. American Economic Review, 91(1), 79–98. https://doi.org/10/cfd4pb
Bernoulli, D. (1954). Exposition of a New Theory on the Measurement of Risk. Econometrica, 22(1), 23–36. https://doi.org/10/cw729v (Original work published 1738)
Bettis, R. A. (2017). Organizationally Intractable Decision Problems and the Intellectual Virtues of Heuristics. Journal of Management, 43(8), 2620–2637. https://doi.org/10/gf2r6w
Bower, J. L. (1970). Managing the resource allocation process: A study of corporate planning and investment. Division of Research, Graduate School of Business Administration, Harvard University.
Bower, J. L., & Gilbert, C. G. (Eds.). (2005). From resource allocation to strategy. Oxford University Press.
Boyd, T. (2021, June 3). Wesfarmers CEO proves his worth. Australian Financial Review. https://www.afr.com/chanticleer/wesfarmers-ceo-proves-his-worth-20210603-p57xmq
Bradley, D. (2013). Decision Theory, Philosophical Perspectives. In H. E. Pashler (Ed.), Encyclopedia of the mind. SAGE Publications, Inc. https://doi.org/10.4135/9781452257044.n81
Bukszar, E., & Connolly, T. (1988). Hindsight Bias and Strategic Choice: Some Problems in Learning From Experience. Academy of Management Journal, 31(3), 628–641. https://doi.org/10/gjscqv
Burgelman, R. A. (1983). A Process Model of Internal Corporate Venturing in the Diversified Major Firm. Administrative Science Quarterly, 28(2), 223–244. https://doi.org/10/dsvjgf
Camerer, C., & Lovallo, D. (1999). Overconfidence and Excess Entry: An Experimental Approach. American Economic Review, 89(1), 306–318. https://doi.org/10/bnkbkb
Cavagnac, M. (2005). Strategic managerial incentives under adverse selection. Managerial and Decision Economics, 26(8), 499–512. https://doi.org/10/cwdbtp
Chen, M. K., Lakshminarayanan, V., & Santos, L. R. (2006). How Basic Are Behavioral Biases? Evidence from Capuchin Monkey Trading Behavior. Journal of Political Economy, 114(3), 517–537. https://doi.org/10/fr7jw7
Christensen-Szalanski, J. J. J., & Willham, C. F. (1991). The hindsight bias: A meta-analysis. Organizational Behavior and Human Decision Processes, 48(1), 147–168. https://doi.org/10/fhnf22
Coase, R. H. (1937). The Nature of the Firm. Economica, 4(16), 386–405. https://doi.org/10/fnm876
Cyert, R. M., Simon, H. A., & Trow, D. B. (1956). Observation of a Business Decision. The Journal of Business, 29(4), 237–248. https://doi.org/10/fm8x9b
Das, T. K., & Teng, B.-S. (1999). Cognitive Biases and Strategic Decision Processes: An Integrative Perspective. Journal of Management Studies, 36(6), 757–778. https://doi.org/10/c84dxp
Davis, P. S., Robinson, R. B., Pearce, J. A., & Park, S. H. (1992). Business unit relatedness and performance: A look at the pulp and paper industry. Strategic Management Journal, 13(5), 349–361. https://doi.org/10/bx3wpx
Einhorn, H. J., & Hogarth, R. M. (1987). Decision making: Going forward in reverse. Harvard Business Review, 65(1), 66–70. https://hbr.org/1987/01/decision-making-going-forward-in-reverse
Friedman, M., & Savage, L. J. (1948). The Utility Analysis of Choices Involving Risk. Journal of Political Economy, 56(4), 279–304. https://doi.org/10.1086/256692
Galesic, M., & Garcia-Retamero, R. (2010). Statistical Numeracy for Health: A Cross-cultural Comparison With Probabilistic National Samples. Arch Intern Med, 170(5), 462–468. https://doi.org/10/fmj7q3
Garbuio, M., & Lovallo, D. (2017). Does organizational politics kill company growth? Review of International Business and Strategy, 27(4), 410–433. https://doi.org/10/gjscsw
Gavetti, G., Levinthal, D. A., & Rivkin, J. W. (2005). Strategy making in novel and complex worlds: The power of analogy. Strategic Management Journal, 26(8), 691–712. https://doi.org/10/b64gsr
Gentner, D. (1983). Structure-Mapping: A Theoretical Framework for Analogy. Cognitive Science, 7(2), 155–170. https://doi.org/10/dw52z8
Gentner, D., & Markman, A. B. (1997). Structure mapping in analogy and similarity. American Psychologist, 52(1), 45–56. https://doi.org/10/fm4rrb
Gentner, D., Rattermann, M. J., & Forbus, K. D. (1993). The Roles of Similarity in Transfer: Separating Retrievability From Inferential Soundness. Cognitive Psychology, 25(4), 524–575. https://doi.org/10/b7tzks
Gertner, R. H., Scharfstein, D. S., & Stein, J. C. (1994). Internal Versus External Capital Markets. The Quarterly Journal of Economics, 109(4), 1211–1230. https://doi.org/10.2307/2118361
Gigerenzer, G. (2008). Why Heuristics Work. Perspectives on Psychological Science, 3(1), 20–29. https://doi.org/10/d8h4qv
Graham, J. R., & Harvey, C. R. (2001). The theory and practice of corporate finance: Evidence from the field. Journal of Financial Economics, 60(2), 187–243. https://doi.org/10/fpdzrj
Graham, J. R., Harvey, C. R., & Puri, M. (2015). Capital allocation and delegation of decision-making authority within firms. Journal of Financial Economics, 115(3), 449–470. https://doi.org/10/gfvz8d
Grant, R. M., & Jammine, A. P. (1988). Performance differences between the wrigley/rumelt strategic categories. Strategic Management Journal, 9(4), 333–346. https://doi.org/10/fdh8xm
Haigh, M. S., & List, J. A. (2005). Do Professional Traders Exhibit Myopic Loss Aversion? An Experimental Analysis. The Journal of Finance, 60(1), 523–534. https://doi.org/10/c7jn9k
Harbaugh, W. T., Krause, K., & Vesterlund, L. (2001). Are adults better behaved than children? Age, experience, and the endowment effect. Economics Letters, 70(2), 175–181. https://doi.org/10/db826t
Harrison, J. S., Hall, E. H., & Nargundkar, R. (1993). Resource Allocation as an Outcropping of Strategic Consistency: Performance Implications. Academy of Management Journal, 36(5), 1026–1051. https://doi.org/10/gjscqw
Haselton, M. G., Bryant, G. A., Wilke, A., Frederick, D. A., Galperin, A., Frankenhuis, W. E., & Moore, T. (2009). Adaptive Rationality: An Evolutionary Perspective on Cognitive Bias. Social Cognition, 27(5), 733–763. https://doi.org/10/c49fjq
Horn, J. T., Lovallo, D. P., & Viguerie, S. P. (2005). Beating the odds in market entry. McKinsey Quarterly, 4. https://www.mckinsey.com/business-functions/strategy-and-corporate-finance/our-insights/hidden-flaws-in-strategy
Kahneman, D. (2003). Maps of Bounded Rationality: Psychology for Behavioral Economics. The American Economic Review, 93(5), 1449–1475. https://doi.org/10/b5gfrt
Kahneman, D., & Lovallo, D. (1993). Timid Choices and Bold Forecasts: A Cognitive Perspective on Risk Taking. Management Science, 39(1), 17–31. https://doi.org/10/c8vntn
Kahneman, D., & Tversky, A. (1979). Prospect Theory: An Analysis of Decision under Risk. Econometrica, 47(2), 263–291. https://doi.org/10/g98
Kokkinis, A. (2019). Exploring the effects of the ’bonus cap’ rule: The impact of remuneration structure on risk-taking by bank managers. Journal of Corporate Law Studies, 19(1), 167–195. https://doi.org/10/gjspz9
Koller, T., Lovallo, D., & Williams, Z. (2012). Overcoming a bias against risk. McKinsey Quarterly, 4. https://www.mckinsey.com/business-functions/strategy-and-corporate-finance/our-insights/overcoming-a-bias-against-risk
Konold, C., Pollatsek, A., Well, A., Lohmeier, J., & Lipson, A. (1993). Inconsistencies in Students’ Reasoning about Probability. Journal for Research in Mathematics Education, 24(5), 392. https://doi.org/10/bq4hvm
Lang, L. H. P., & Stulz, R. M. (1994). Tobin’s q, Corporate Diversification, and Firm Performance. Journal of Political Economy, 102(6), 1248–1280. https://doi.org/10/dgjvg3
Langer, E. J. (1975). The illusion of control. Journal of Personality and Social Psychology, 32(2), 311–328. https://doi.org/10/bhghpg
Liebeskind, J. P. (2000). Internal Capital Markets: Benefits, Costs, and Organizational Arrangements. Organization Science, 11(1), 58–76. https://doi.org/10/dkmq6t
Liu, B., & McConnell, J. J. (2013). The role of the media in corporate governance: Do the media influence managers’ capital allocation decisions? Journal of Financial Economics, 110(1), 1–17. https://doi.org/10/gffggq
Lovallo, D., & Kahneman, D. (2003). Delusions of Success: How Optimism Undermines Executives’ Decisions. Harvard Business Review, 81(7).
Lovallo, D., Koller, T., Uhlaner, R., & Kahneman, D. (2020). Your Company Is Too Risk-Averse. Harvard Business Review, 98(2), 104–111.
Lubatkin, M., & Shrieves, R. E. (1986). Towards Reconciliation of Market Performance Measures to Strategic Management Research. Academy of Management Review, 11(3), 497–512. https://doi.org/10/ftmktj
Mannes, A. E., & Moore, D. A. (2013). A Behavioral Demonstration of Overconfidence in Judgment. Psychological Science, 24(7), 1190–1197. https://doi.org/10/f443q6
Markowitz, H. (1952). Portfolio Selection. The Journal of Finance, 7(1), 77–91. https://doi.org/10/bhzd
Mazzolini, R. (1981). How strategic decisions are made. Long Range Planning, 14(3), 85–96. https://doi.org/10/dm8852
McCray, G. E., Purvis, R. L., & McCray, C. G. (2002). Project Management under Uncertainty: The Impact of Heuristics and Biases. Project Management Journal, 33(1), 49–57. https://doi.org/10/gjscsr
McKenzie, C. R. M., Liersch, M. J., & Yaniv, I. (2008). Overconfidence in interval estimates: What does expertise buy you? Organizational Behavior and Human Decision Processes, 107(2), 179–191. https://doi.org/10/b22rw7
Meissner, P., Sibony, O., & Wulf, T. (2015). Are you ready to decide? McKinsey Quarterly. https://www.mckinsey.com/business-functions/strategy-and-corporate-finance/our-insights/are-you-ready-to-decide
Mintzberg, H., Raisinghani, D., & Théorêt, A. (1976). The Structure of "Unstructured" Decision Processes. Administrative Science Quarterly, 21(2), 246–275. https://doi.org/10/b2t8ks
Mintzberg, H., & Waters, J. A. (1985). Of strategies, deliberate and emergent. Strategic Management Journal, 6(3), 257–272. https://doi.org/10/bs2pm3
Noda, T., & Bower, J. L. (1996). Strategy making as iterated processes of resource allocation. Strategic Management Journal, 17(S1), 159–192. https://doi.org/10/brg23k
Ortner, J., Velthuis, L., & Wollscheid, D. (2017). Incentive systems for risky investment decisions under unknown preferences. Management Accounting Research, 36, 43–50. https://doi.org/10/gbz24d
Parayre, R. (1995). The strategic implications of sunk costs: A behavioral perspective. Journal of Economic Behavior & Organization, 28(3), 417–442. https://doi.org/10/d2wg72
Pascal, B. (1999). Pensées and other writings (A. Levi, Ed.; H. Levi, Trans.). Oxford University Press. (Original work published 1670)
Penrose, E. (2009). The Theory of the Growth of the Firm (4. ed., rev. ed). Oxford University Press. http://books.google.com?id=zCAUDAAAQBAJ (Original work published 1959)
Porter, M. E. (1980). Competitive Strategy: Techniques for Analyzing Industries and Competitors. Free Press. http://books.google.com?id=sDSxAQAACAAJ
Porter, M. E. (1985). Competitive Advantage: Creating and Sustaining Superior Performance. Free Press. http://books.google.com?id=7UqQXsQ_dj4C
Porter, M. E. (1987). From competitive advantage to corporate strategy. Harvard Business Review, 65(3), 43–43. https://hbr.org/1987/05/from-competitive-advantage-to-corporate-strategy
Powell, T. C., Lovallo, D., & Fox, C. R. (2011). Behavioral strategy. Strategic Management Journal, 32(13), 1369–1386. https://doi.org/10/d8m8pw
Puri, M., & Robinson, D. T. (2007). Optimism and economic choice. Journal of Financial Economics, 86(1), 71–99. https://doi.org/10/c9839j
Rajan, R., Servaes, H., & Zingales, L. (2000). The Cost of Diversity: The Diversification Discount and Inefficient Investment. The Journal of Finance, 55(1), 35–80. https://doi.org/10/bdsh6h
Reichelstein, S. (1997). Investment Decisions and Managerial Performance Evaluation. Review of Accounting Studies, 2(2), 157–180. https://doi.org/10/fp2zqc
Remer, D. S., Stokdyk, S. B., & Van Driel, M. (1993). Survey of project evaluation techniques currently used in industry. International Journal of Production Economics, 32(1), 103–115. https://doi.org/10/bsc6bs
Roxburgh, C. (2003). Hidden flaws in strategy. McKinsey Quarterly, 2. https://www.mckinsey.com/business-functions/strategy-and-corporate-finance/our-insights/hidden-flaws-in-strategy
Ruggeri, K., Alí, S., Berge, M. L., Bertoldo, G., Bjørndal, L. D., Cortijos-Bernabeu, A., Davison, C., Demić, E., Esteban-Serna, C., Friedemann, M., Gibson, S. P., Jarke, H., Karakasheva, R., Khorrami, P. R., Kveder, J., Andersen, T. L., Lofthus, I. S., McGill, L., Nieto, A. E., … Folke, T. (2020). Replicating patterns of prospect theory for decision under risk. Nature Human Behaviour, 4(6, 6), 622–633. https://doi.org/10/ggwrdh
Rumelt, R. P. (1974). Strategy, Structure, and Economic Performance. Division of Research, Graduate School of Business Administration, Harvard University. http://books.google.com?id=f1WqAAAAIAAJ
Schaefer, R. E., & Borcherding, K. (1973). The assessment of subjective probability distributions: A training experiment. Acta Psychologica, 37(2), 117–129. https://doi.org/10/dpzkfb
Schwenk, C. R. (1984). Cognitive simplification processes in strategic decision-making. Strategic Management Journal, 5(2), 111–128. https://doi.org/10/b2hsgw
Sengul, M., Costa, A. A., & Gimeno, J. (2019). The Allocation of Capital within Firms. Academy of Management Annals, 13(1), 43–83. https://doi.org/10/gfgvqj
Shapira, Z., & Shaver, J. M. (2014). Confounding changes in averages with marginal effects: How anchoring can destroy economic value in strategic investment assessments. Strategic Management Journal, 35(10), 1414–1426. https://doi.org/10/gkhfb3
Shelton, L. M. (1988). Strategic business fits and corporate acquisition: Empirical evidence. Strategic Management Journal, 9(3), 279–287. https://doi.org/10/fbhcgd
Simon, H. A. (1955). A Behavioral Model of Rational Choice. The Quarterly Journal of Economics, 69(1), 99. https://doi.org/10/dw3pfg
Soll, J. B., & Klayman, J. (2004). Overconfidence in Interval Estimates. Journal of Experimental Psychology: Learning, Memory, and Cognition, 30(2), 299–314. https://doi.org/10/dtpsvd
Staw, B. M. (1981). The Escalation of Commitment To a Course of Action. Academy of Management Review, 6(4), 577–587. https://doi.org/10/fdqtjg
Swalm, R. O. (1966). Utility Theory–Insights into Risk Taking. Harvard Business Review, 44(6), 123–136.
Teece, D. J. (1982). Towards an economic theory of the multiproduct firm. Journal of Economic Behavior & Organization, 3(1), 39–63. https://doi.org/10/bdwvvq
Teece, D. J. (1980). Economies of scope and the scope of the enterprise. Journal of Economic Behavior & Organization, 1(3), 223–247. https://doi.org/10/fxcj3r
Thamhain, H. J. (2014). Assessing the Effectiveness of Quantitative and Qualitative Methods for R&D Project Proposal Evaluations. Engineering Management Journal, 26(3), 3–12. https://doi.org/10/gjsm2q
Tom, S. M., Fox, C. R., Trepel, C., & Poldrack, R. A. (2007). The Neural Basis of Loss Aversion in Decision-Making Under Risk. Science, 315(5811), 515–518. https://doi.org/10/bhtqgn
Tversky, A., & Kahneman, D. (1974). Judgment under Uncertainty: Heuristics and Biases. Science, 185(4157), 1124–1131. https://doi.org/10/gwh
Tversky, A., & Kahneman, D. (1992). Advances in prospect theory: Cumulative representation of uncertainty. Journal of Risk and Uncertainty, 5(4), 297–323. https://doi.org/10/cb57hk
Ullrich, M. J., & Tuttle, B. M. (2004). The Effects of Comprehensive Information Reporting Systems and Economic Incentives on Managers’ Time-Planning Decisions. Behavioral Research in Accounting, 16, 89–105. https://doi.org/10/b99r4n
Vivalt, E., & Coville, A. (2021). How Do Policy-Makers Update Their Beliefs? (p. 51). http://evavivalt.com/wp-content/uploads/How-Do-Policymakers-Update.pdf
von Holstein, C.-A. S. S. (1971). Two techniques for assessment of subjective probability distributions — An experimental study. Acta Psychologica, 35(6), 478–494. https://doi.org/10/fgg6jn
von Neumann, J., Morgenstern, O., & Rubinstein, A. (1944). Theory of Games and Economic Behavior. Princeton University Press. http://www.jstor.org/stable/j.ctt1r2gkx
Wainberg, J. S. (2018). Stories vs Statistics: The Impact of Anecdotal Data on Managerial Decision Making. In Advances in Accounting Behavioral Research (Vol. 21, pp. 127–141). Emerald Publishing Limited. https://doi.org/10.1108/S1475-148820180000021006
Weber, E. U., & Hsee, C. (1998). Cross-Cultural Differences in Risk Perception, but Cross-Cultural Similarities in Attitudes Towards Perceived Risk. Management Science, 44(9), 1205–1217. https://doi.org/10/c4rqqd
Wernerfelt, B. (1984). A Resource-Based View of the Firm. Strategic Management Journal, 5(2), 171–180. https://doi.org/10/cm664p
Wernerfelt, B., & Montgomery, C. A. (1988). Tobin’s q and the Importance of Focus in Firm Performance. The American Economic Review, 78(1), 246–250. http://www.jstor.org/stable/1814713
Williamson, O. E. (1981). The Economics of Organization: The Transaction Cost Approach. American Journal of Sociology, 87(3), 548–577. https://doi.org/10/bqzkpb
Yates, J. F., Jagacinski, C. M., & Faber, M. D. (1978). Evaluation of partially described multiattribute options. Organizational Behavior and Human Performance, 21(2), 240–251. https://doi.org/10/br3dbv